- The Bank
- People
- All Services
- Private Banking
- Savings and Investment
- GoBulling Platforms
- Institutional and Corporate
- Insights
- Login My.BancoCarregosa
- Contacts
Enter your Username to gain access to your Bank. Complete your authentication on the next screen.
If you are not yet a client, open your account here or contact us for more information
How machine learning is changing asset management
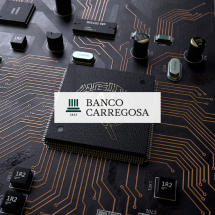
Machine learning is an important tool in asset management, powered by human experience. Find out how to get the best of both worlds when building a portfolio.
Although Artificial Intelligence (AI) has been around since 1950, it is only recently that the growth in processing power, cloud storage and the proliferation of data has brought this technology to the centre of discussion. Asset management is no exception. In particular, Machine Learning presents itself as one of the innovations with the greatest potential to redesign the performance of financial managers around the world.
To put the scale of the amount of data are currently available to investors into context, we need only look at one specific data set that is a key component of the modern investment process: public financial information from companies. All publicly traded companies must report financial information, including reports and accounts, legal proceedings or property records. For the Russell 3000 index alone, which comprises around 3,000 of the largest U.S. companies by market capitalisation, that means more than 12,000 documents in a given fiscal year.
Processing all this information to extract insights, patterns and outliers requires intensive use of technology. But to really make decisions from these datasets, you need the monitoring and supervision of a specialised team that can guide investors. So how important is technology in general, and Machine Learning in particular, to asset management? And how can investors get the best out of these two worlds, cutting-edge technology and human intervention?
The role of technology in asset management
The use of technology in asset management is far from being something new, whether in trading, risk management, operations or customer services. As an example, Banco Carregosa was the first financial institution to offer an online brokerage service in Portugal, in 2000, when smartphones were merely a mirage.
As technology continues to evolve and computing power increases, the scope of digital intervention grows and new purposes for action are identified. Artificial Intelligence and Machine Learning are two of the leading examples of this growth, directly applicable to asset management.
However, they are also buzzwords widely used in different contexts, which can cause confusion among investors. In a financial context, the big contribution of AI and Machine Learning relates to the ability to classify large data sets and process information. To what end? To identify patterns and outliers.
Machine Learning thus has wide-ranging applications in financial services, which can bring benefits to consumers, businesses and the economy. For example, many banks use this technology to prevent money laundering. For consumers, it helps reduce the number of wrongly blocked payments. For banks, it frees up scarce resources and speeds up internal processes. For the economy as a whole, it can help banks and authorities to more accurately identify illicit financial activity.
Machine Learning also has the potential to provide more inclusive and personalised products to consumers. For example, it is already used by banks and businesses around the world to provide risk assessments for individuals with limited credit history, which can help them access financial services.
Credit rating agencies are including Machine Learning in consumer credit scores, including the money they earn and spend, the taxes they pay, the savings they have and the investments they make.
The use of Machine Learning in the financial ecosystem is booming, and has not been affected by the pandemic, as a recent study carried out by the Bank of England shows.
THE USE OF MACHINE LEARNING IN THE FINANCIAL ECOSYSTEM (2020)
Source: Bank of England
Our vision, which we have held for over 180 years, is that the use of technology is essential for improving data quality and analysis for decision-making and mitigating risk. It is also essential to deliver a competitive advantage to our clients. However, there is still a number of challenges to its dissemination in asset management.
The challenges of technology in asset management
One of the main obstacles has to do with the very nature of data. Market data is "noisy". What this means is that the data on which the algorithms are based is subject to impacts from external sources, remote and rare but drastic events with knock-on impacts that are difficult to quantify.
On the other hand, data is easily skewed by the public and managers. Taking decisions based on this information would only amplify a widespread fear or high-spirited optimism based on unfounded assumptions.
The Bank's long history allows us to have a more discerning perspective in the processing of information. As we all know, past data is scarce, i.e. data from previous decades is less robust than the data we have today. The rise of quantum and passive investments has only compounded this problem: non-human decision-making leads to decisions that appear jumbled in market data sets.
On the other hand, if left to their own devices, Machine Learning algorithms tend to force correlations, and find cause-and-effect links and patterns that any experienced investor would disregard. Not to mention the difficulty in introducing fundamental investment principles and values into decision making. How do you teach an algorithm to be heedful of sustainability or not to collaborate with oppressive regimes? So far, no one knows the answers to these questions, not even the most learned quantum fund managers.
The ability of technology to analyse vast amounts of data in seconds is impressive, but it is human experience that brings this power to life. Machine Learning algorithms can analyse terabytes of data in a microsecond and generate insights, they can determine relationships between large numbers of data inputs in a way that seemed impossible just a few years ago. Although the computational power of Machine Learning exceeds that of humans, the success of its application depends on the manager's expertise to develop and refine it.
The best of both worlds: technology combined with human experience
There is a big difference between letting a Machine Learning algorithm dictate the ads we see on Facebook and allowing it to make investment decisions for us. For a fund manager, the consequences of acting on "buzz” rather than relevant information are drastic.
To take advantage of Machine Learning, Banco Carregosa employees are encouraged to think outside the black box of algorithms, to question the data based on experience and to form an opinion based on very diverse credible sources of information. For us, Machine Learning is a part of a larger ecosystem in which an experienced team takes decisions using the conclusions generated through technology.
The very structure of Banco Carregosa is a reflection of this, which takes into account the interaction between people and technology, in the form of an experienced Investment Committee. The purpose of the Investment Committee is to guide the various stakeholders in a concerted manner to make the best decisions. It is formed by the most experienced members of the bank, who identify and discuss the major economic, social, technological, political, monetary and fiscal trends likely to shape investment decisions in a medium and long term time horizon.
Our investment selection process follows a set of well-established, time-tested steps that have survived times of uncertainty, such as the financial and sovereign crises of 2008 and 2012.
A forward-looking investment approach for 180 years
"A good decision is based on knowledge, not numbers". When Plato wrote this sentence almost 2,500 years ago, he hardly imagined it would be used in an asset management or Machine Learning context.
Machine Learning plays a major role in financial decision-making and its importance will continue to grow. It is especially useful in a context where the asset manager can quickly test hypotheses based on data, process information from different sources and build alternative scenarios.
It allows us to analyse portfolio data and accurately identify significant behavioural patterns that lead to measurable performance improvement. This high computing power is then contrasted with 'real world' experience, and discussed amongst a professional investment team, to ensure the best results for our clients.
For over 180 years we have been guiding our approach and investment philosophy towards the future. Get in touch with our team, so we can match the best of technology with the irreplaceable experience of our managers, in a customised relationship.
Click here for additional Legal Information.